New Report on AI Topics Categorization
Read the outcome of our presentation of the AI topics categorization at the EBDVF 2022 in Prague
VISION is a proud sponsor and participant of the European Big Data Value Forum 2022 in Prague. On 22 November 2022, we hosted a VISION session titled “A Roadmap for Trustworthy European AI”. In this session, a follow-up to the 2nd ICT-48 Community Workshop, we presented our initial AI topics categorization to the broader data & AI community and collected feedback.
The session started with Joachim de Greeff explaining the goal of VISION and our task at hand — to support the integrated roadmapping towards trustworthy European AI. To get to a shared SRA, Vision is conducting a mapping exercise to get a sense of what is happening in Europe with regard to AI research. The objective of the VISION session at EBDVF was to gather input and ideas from the broader community. Around ca. 40 participants from all across Europe – mainly in research and industry (a 50-50 mix) with a few people from public administrations – shared their feedback on the first draft of a topic categorization.
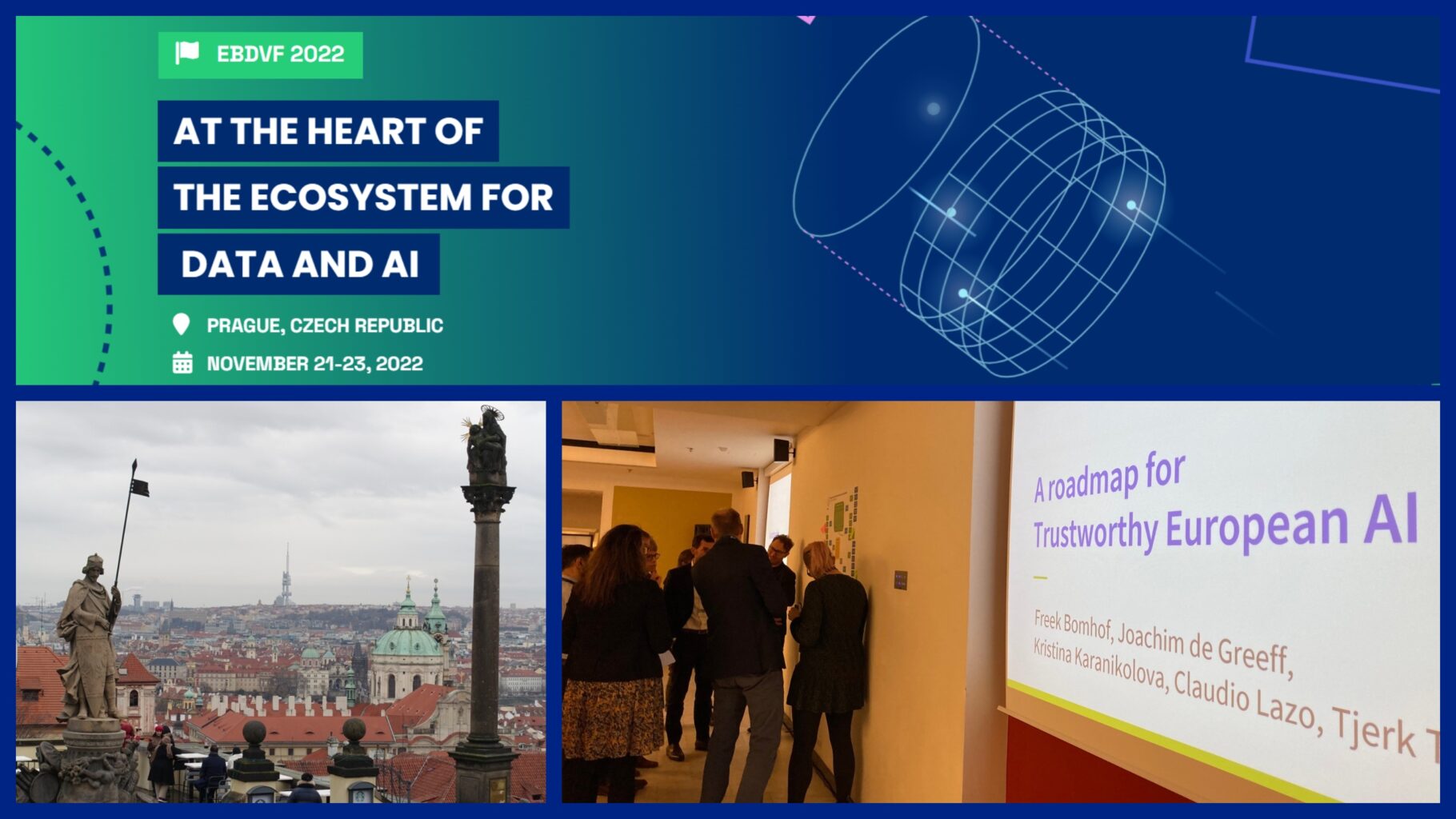
AI topics categorization
Freek Bomhof explained VISION’s work on the AI topics categorization so far. Besides the categorization itself, we highlighted how it fits into the bigger picture, our approach and the used sources. The results (what fitted well, what didn’t?), and the lessons learned. This information was also presented in the 2nd ICT-48 community workshop and can be found in the report.
Next, Kristina Karanikolova surveyed the participants on which topics they suggested adding; who they considered the main target audience; and the value of such a categorization. Participants were invited to add their favorite (or any missing) topics to the categorization, and provided several topics such as verifiable AI, trustworthy AI or federated learning, most of which we were able to identify in the categorization.
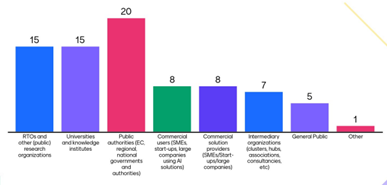
The participants found the main target audience for such a categorization to be public authorities. After that, knowledge and research institutes (academia, RTO’s, public research organizations), and in third place are commercial users, providers and intermediaries. Finally, there was some interest in usage by the general public.
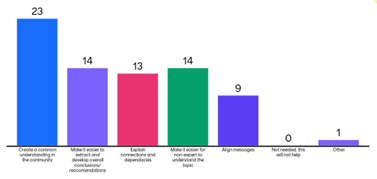
The main way in which such a categorization can help is creating a common understanding in the community. After that, it makes it easier for non-experts to understand the topic and extract and develop overall conclusions. Finally, it helps AI stakeholders to align their messages.
Results from the brainstorming sessions
Several themes came to light within the discussions (in break-out groups) on the categorization : 1) the objective, approach and audience; 2) The form and structure; 3) AI topics that were missing or unclear.
Specify exactly the objective, audience and approach for the categorization
The categorization should be fit for purpose, and not an ontology for the sake of being one. Therefore it is relevant to define exactly the objective and who the audience is. The customer requirements will depend on factors such as organization type (RTO, academia) and application domain. Moreover, in the definition of ‘human-centric AI’, the question arises who the central AI user is and there is a difference between a professional user and a citizen or consumer being subject to AI.
As the AI research & innovation ecosystem is a socio-technical system, there might be best practices from socio-technical systems literature (structures/approaches/categorizations) that work in that area as well. These best practices may help address how we validate the categorization (e.g. through literature and domain-specific approaches).
The form and structure elements are essential considerations
Taking the categorization at face value, it is essentially a conceptual high-level overall view. We noticed that both the use of color and level of depth are very important as people interpret the alternating colors as having a meaning, and/or indicating a hierarchy. At lower levels of depth, the AI topics and their subtopics should be defined adequately, as the level of granularity for the categorization is important (e.g. ‘Machine Learning’ can be more granular, as well as ‘trustworthiness’, or more modal).
Moreover, the categorization can be different for each application area (definitions, structures, maturity levels) with different views, ie. highlighting different connections for different views/audiences (researchers, policy makers etc).
Think about how the connection between research and policy topics is made
We also reflected on the structure of the categorization: The connection between the left and right side may be made clearer. There was quite a big discussion about why there is a big separation between the research (left side) and policy part (right side). There are many more cross-connections than can be drawn, as there is an inherent technical and non-technical side to many categories (e.g. trustworthy AI, fairness, explainable AI) – being both a research topic and a policy/funding topic. Moreover, is it wise to separate technological-from non-technological elements so clearly? (Because that is often where problems start!).
One profound question became clear: “how to cluster these topics?”. Currently, there is a thematic clustering, but another option is to cluster the topics into methodologies and outputs or to separate the science and technology topics from the policy topics. Some suggestions were made for acknowledging this: dividing topics into methodologies and outcomes, type of high-level goal, or application domains. These horizontals tie together various aspects that are now classified into different groups.
Topics are more than just a name
For each topic, a rationale can help with the storyline. A rationale would describe why it is selected, where we want to go, tools and methodologies to reach the topics might, what the risks are, and what the challenges are to get there. Progress in these topics can be indicated by the level of excellence or development/maturity. These are different per application domain. You may want to use Technology Readiness Levels (TRL) or Societal Readiness Levels (SRL) for this.
The categorization was strengthened with more AI topics
Several topics were suggested for the categorization. Some of the elements mentioned as missing were related to business models and capturing value, the role of open source for trustworthy AI, and more fine-grained topics (ML, visuals, privacy separate from trustworthiness, etc.).
Core and transversal AI topics
Some topics were introduced, such as one shot/few shot learning, federated learning, decentralized AI, and privacy-preserving NLP as well as more modalities of perception (audio, video, olfactory…). Under AI services, horizontal (research) topics like business models, and the distribution of value by AI can be added.
AI ethics
Trustworthy and ethical AI should be further specified into ethical principles with topics such as privacy, transparency, reliability, verifiability, accountability, etc. while it is also a (research) topic in itself. Moreover, as was mentioned, the topic of Philosophy of AI can be extended to other disciplines in the humanities and social sciences (e.g. sociology of AI), as well as philosophical AI topics (e.g. Strong/general intelligence).
Ecosystem of Excellence
Some additional terms were added or specified such as in the area of oversight and education. The topic of Education and skills can be expanded with upskilling or reskilling of the existing workforce (e.g. a major goal of the Digital Europe Programme), teaching soft skills such as critical engagement, and the use of EdTech.
In providing the technical infrastructure for AI, data is not yet well-represented in the categorization. For instance, data sources, data visualization and data sharing (e.g. data spaces, data marketplaces), digital sovereignty, ownership and control on data (e.g. licensing), as well as synthetic data.
Under innovation & transfer, accelerated adoption of AI-based solutions, specifically in the public sector by innovation sandboxes, and specifically for SMEs by engaging with (European) Digital Innovation Hubs. And the question was raised ‘what metrics are there for AI adoption?’
Ecosystem of Trust
In creating trust among citizens, demystification also consists of ‘debunking AI myths’ (e.g. with publications) and explanations. Governance should be further explored in terms of policy, decision making, regulation, funding, direction, using toolkits and guidelines. Certification is one of the enforcement governance and tools.
Conclusion
The workshop format, where participants stood in smaller groups around a big poster, tasked to comment with post-its, worked really well in generating many additions to the AI topics categorization structure in a short amount of time.
The main insights were:
- To specify exactly the objective, audience and the approach for the categorization, using best practices.
- The form and structure elements (color, depth, granularity, views) are essential considerations. The topic rationale is important, and there are implications to the way in which connections between topic categories are presented.
- The categorization was strengthened with more AI topics across the board: core and transversal AI topics, AI ethics topics, Ecosystem of Excellence, Ecosystem of Trust.
VISION will continue working on this AI topics categorization, trying to include as much as possible the results. We will work on this with the AI Networks of Excellence that are developing their own Strategic Research Agendas (SRA) and together joining them into a European AI SRA, by testing whether we can provide an adequate structure for their SRAs. When it is successful we wish to expand it so that other networks in Europe can use it. The point in the horizon is a joined European AI SRA that uses a common language.