European AI Trend Radar: Summary & Overview
European AI Trend Radar is a compilation that synthesises the insights and discussion from the Theme Development Workshops of TAILOR, HumanE AI Net, VISION and CLAIRE AISBL. These workshops were forums where key players from various AI-related industry sectors and academic fields articulated strategic research directions in AI in Europe. The core of the European AI Trend Radar report is based on the discussions held during these workshops, providing it with a distinctive, ground-up perspective on trends within Europe’s AI landscape. To augment and validate the trends identified in the TDWs, a literature and market search was conducted. This approach ensures that the findings not only reflect expert opinions but are also confirmed by existing academic and industry research, increasing the reliability of the conclusions drawn. However, it is important to highlight that the core of the European AI Trend Radar remains connected to the Theme Development Workshops. This connection offers a NoEs-specific perspective, emphasising practical, real-world applications and innovations in AI that are specifically relevant to Europe.
Two main categories of trends are identified: Broad Trends and Specific Trends. Broad Trends, such as Education, Data, and Trustworthy AI, serve as fundamental elements that impact multiple sectors and guide the overall development of AI in Europe. In contrast, Specific Trends offer focused, actionable insights tailored to particular areas like Research-Industry Collaboration, Procurement Strategies, and the creation of Algorithm Registers. These Specific Trends support more targeted strategies for implementing and integrating AI technologies in specific contexts, enabling customized approaches to promoting AI advancement in Europe. Below, a selection of these key trends is listed and explained in further detail.
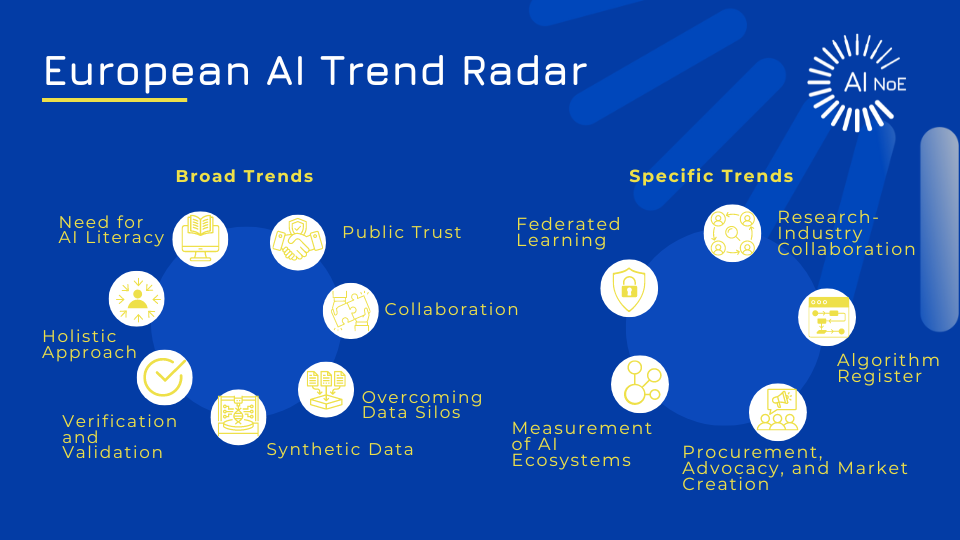
Broad Trends
Education and Communication
Need for AI Literacy
A critical need for tailored educational programs was identified to bridge the gap between technical developers and end-users, particularly in sectors like healthcare, manufacturing, and public administration. For example, AI education in healthcare aims to help professionals understand AI’s potential and limitations, thereby fostering trust and improving patient outcomes.
Public Trust
The workshops emphasized the importance of effective communication strategies to build public trust in AI technologies. For instance, informing the public about AI’s role in healthcare through transparent communication can lead to better acceptance and utilization of AI-driven solutions.
Collaboration
Interdisciplinary research and collaboration, such as between AI experts and public sector workers, are essential for integrating AI into various sectors. This was seen in the energy sector, where AI competency courses were recommended to address gaps in education and promote knowledge transfer.
Data
Overcoming Data Silos
A major challenge highlighted was the need to overcome information silos across organizations to enable better data sharing. For example, in healthcare, a European-wide data infrastructure could advance research by providing access to large, diverse datasets.
Synthetic Data
The use of synthetic data was discussed as a solution for environments where real-world data is scarce, such as in manufacturing where defects are rare but critical for training AI models.
Trustworthy AI
Holistic Approach
Trustworthy AI was identified as crucial, encompassing aspects like robustness, security, explainability, and ethics. For example, AI systems in manufacturing must be robust to changing work conditions, ensuring reliable performance.
Verification and Validation
Ensuring AI systems are safe and reliable, particularly in critical environments like space missions, requires advanced verification techniques. This is critical in high-stakes industries where failure can have catastrophic consequences.
Specific Trends
Measurement of AI Ecosystems
The need for systematic approaches to measure the impact of AI on society was emphasized, including evaluating AI’s societal and economic effects. For instance, in manufacturing, understanding how AI affects productivity can guide ethical and effective AI integration.
Research-Industry Collaboration
The TDWs highlighted the importance of joint labs and collaboration between academia and industry. For example, in manufacturing, joint labs can accelerate the practical application of AI, bridging the gap between research and real-world industrial needs.
Procurement, Advocacy, and Market Creation
Governments play a significant role in shaping AI markets through procurement and market creation. Strategic decisions between in-house development and private procurement can stimulate innovation. The TDWs discussed how public-private partnerships and updated procurement guidelines can enhance AI adoption, especially in sectors like healthcare.
Algorithm Register
The creation of algorithm registers was identified as a way to enhance transparency and accountability in AI systems. This would involve documenting AI algorithms used in systems, making them visible and open to scrutiny. This is crucial for building trust, particularly in sectors where AI decisions have significant consequences.
Federated Learning
Federated Learning (FL) was highlighted as a promising approach in manufacturing, allowing the training of AI models on partial datasets across multiple locations without sharing raw data. This method aligns with privacy regulations like GDPR and is effective in sectors handling sensitive information.
These trends and examples underscore the importance of a comprehensive approach to AI development, focusing on education, data management, trustworthy AI, collaboration, and strategic government intervention to foster innovation and public trust in AI technologies.
Future Outlook
The future of AI in Europe, as outlined by the European Trend Radar, suggests increasing integration of AI across sectors, driven by transparency, trust, and collaboration. Advancements in AI education and communication will likely bridge the gap between developers and end-users, creating a more AI-literate society. Data management and privacy, particularly through Federated Learning, will become increasingly critical as AI systems handle larger datasets. The focus on Trustworthy AI will evolve into more robust frameworks ensuring ethical and secure systems. Collaboration between academia, industry, and government will remain vital, with joint labs and public-private partnerships accelerating innovation. As AI technologies mature, establishing algorithm registers and systematic performance measurements will be crucial in maintaining accountability and trust, positioning Europe as a leader in ethical AI development.